Like humans, AI systems absorb biases—both overt and subtle—that are baked into their training data or even their fundamental architecture. By understanding the roots of bias in AI models and deploying thoughtful mitigation strategies, finance teams can develop AI tools that make fair, ethical and accurate predictions. This requires an ongoing commitment to inclusion and transparency in the data used to train the AI.
Cast a Wide Net for Representative Data
Models are only as unbiased as their inputs. For instance, a financial forecasting model predominantly trained on bull market data may fail to predict bearish conditions. Sampling bias also creeps in if algorithms designed to assess new ventures are built using data only from large, established companies.
Training data that captures a diversity of scenarios, across market cycles and business types, is essential for balanced AI. Feature selection also plays a key role. For example, an algorithm assessing new ventures should weigh intangible assets like intellectual capital, not just hard financials, to avoid undervaluing a business.
Keep Humans in the Loop, But Check Their Biases Too
While the human element in AI is crucial, it can inadvertently lead to bias in AI models. Our personal views and biases in the financial sector play a role in how we categorize and label financial information, leading to data labeling bias.
On a broader level, societal biases may skew the interpretation of outputs, such as undervaluing emerging market investments due to assumptions. If an AI model designed to assess international investments is training on data primarily sourced from well-established markets, there’s a risk that the AI might assign a lower value to prospects in less developed markets. This could be a result of ingrained societal prejudices or a simple lack of diverse data inclusion. Such situations not only reinforce societal biases but also pose serious ethical dilemmas, especially when these AI evaluations play a role in shaping major financial strategies and investment choices.
Stay Vigilant for Blind Spots
Despite their complexity, AI models cannot foresee unforeseeable events or adapt to abrupt regulatory shifts. Their effectiveness is limited to the quality and scope of their training data. Even with extensive data, uncharted scenarios may arise. Acknowledging and addressing these limitations is crucial for developing AI systems that are equitable, precise, and advantageous.
In addition to AI biases, finance professionals should be aware of additional challenges that require proactive monitoring.
- Overfitting is a key issue where models overly adapt to training data. This results in models that excel in tests using historical data but falter in real-world situations, a phenomenon evident in certain stock forecasting models.
- The “black box” problem, or the lack of explainability, poses another hurdle. It complicates matters for stakeholders who need clarity to interpret AI results. Moreover, the extensive requirements of AI systems, like cost and ongoing management, can burden smaller finance teams.
- Ongoing training is crucial to mitigate model drift. Models can become less effective without continual updates, such as data around current market trends. Model drift can potentially lead to off-target financial forecasts or flawed investment approaches.
Use These Strategies to Reduce Bias in AI Models
- Audit data routinely for completeness, accuracy and representation. Does your data cover all necessary conditions or circumstances?
- Set formal standards for labeling data, ensuring that data labels are diverse enough to reduce bias in AI outputs. When classifying investments, for example, are you labeling investment data as high- versus low-risk?
- Scrutinize model features to ensure diversity and balance. For example, does a cash flow forecasting model incorporate 360 views of receivables, payables, inventory and more?
- Validate models on recent, real-world data—not just historical data. To evaluate a credit risk algorithm effectively, it’s important to test it against recent loan default rates instead of just relying on historical credit scores, ensuring the model stays current and relevant.
- Use automated monitoring to catch anomalies early. For forecasting, regular monitoring can help detect market fluctuations and make it easier to adjust the model.
- Use diverse evaluation metrics, such as accuracy, precision (correct positive identifications), and recall (identification of actual positives), to comprehensively assess AI model performance and ensure its effectiveness in various scenarios.
- Understand the key drivers behind your AI recommendations and remove unnecessary complexity to help increase transparency and explainability.
Prepare Your Data for Success
Creating unbiased AI is a collaborative effort requiring diligence, empathy and a willingness to confront our own limitations. With care and compassion, we can build tools that make ethical, equitable predictions to guide financial decisions. The future success of AI relies on addressing biases today.
Navigating bias in AI can be difficult if you’re unaware of the blind spots in your data. Paro matches businesses with on-demand finance experts to help audit and prepare your data for success. Let us match you with the special skills you need to confront bias at every step of the model pipeline. Schedule a free consultation.
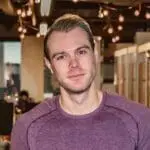
About the Author
Kody Myers, Senior Director of Product at Paro, brings a decade of product management experience fueled by a passion for AI-driven solutions. Kody thrives in the ambiguous environment of early-stage, high-growth startups, developing long-term product and data strategies. His entrepreneurial and financial background, coupled with the analytical rigor developed during his time in market research, positions him at the forefront of AI product innovation in finance.