As AI becomes increasingly embedded in finance and accounting, professionals will share greater responsibility in the training and learning of AI models. The data supplied by finance serves as the lifeblood for AI models. Therefore, accountants, controllers, analysts and CFOs will need to provide quality data and validation to ensure models generate trusted insights. By understanding training fundamentals, finance leaders can better evaluate model relevancy, minimize biases and collaborate cross-functionally.
Learning Is Dependent Upon Data Quality
When training AI models, assumptions can cloud judgments. Finance professionals should remain aware of common misconceptions.
For example, AI detects patterns in historical data, but it cannot predict unexpected events. Models can also miss nuances or disruptive events that humans may be able to detect based on their professional experience and industry knowledge. Unfortunately, supplying AI models with more and more training data does not guarantee better outcomes. Irrelevant data can derail models and lead to poorer outcomes.
By understanding the myths around AI, you can set better expectations around its learning.
Be Meticulous In Data Preparation
The first step in the learning process is data preparation. Data preparation should be handled with the same precision and care as other compliance functions, like financial or tax reporting, in order to get the best results.
For better outcomes, make sure data is:
- Clean: remove inconsistencies, errors and duplicate records to avoid confusing models.
- Normalized: scale and standardize data so models can identify meaningful patterns.
- Augmented: supplement data to fill gaps and improve model training if historical data is limited.
Establish Learning Methods in AI Models
AI models use two different approaches to learning: supervised and unsupervised.
In supervised learning, the model is trained on labeled input-output pairs. It learns the relationship between inputs and outputs with the goal of making accurate predictions.
For example, a CFO wants to forecast future sales. She uses an AI model trained on historical advertising spend (input) and sales data (output). The model analyzes this to understand their relationship. Given new ad spend data, it can then predict expected sales.
In unsupervised learning, the model is given data with no defined input-output pairs. It detects inherent patterns and groupings within the data.
A controller may use an AI model with this learning technique to identify potential fraud. An AI model analyzes large volumes of transaction data, clustering those with similar attributes. Transactions that don’t fit into identified groups are flagged as potential anomalies for further review.
Perform Frequent Model Validation
Rigorous validation is crucial once the AI model is trained. To evaluate accuracy, professionals should compare model suggestions against new data not used in its learning. For example, before relying on an AI model’s stock price predictions, test them against recent market data. This validation safeguards against blind trust in the AI’s insights.
Effective Training Combines Data With Human Expertise
Effective model building starts with quality data. Clean, robust datasets enable accurate insights and efficiencies from AI tools. However, professionals play an equally crucial role in maximizing value. Like any technology, AI’s success depends on human expertise guiding its application.
At Paro, we can help your finance and accounting team prepare for implementation of new systems, shore up data practices and streamline transformation to adapt for the future. Our fractional finance experts bring years of experience in optimizing processes to build business success. Schedule a free consultation to learn more.
About the Author
Eli Gill is the Vice President of Engineering, Product, and AI at Paro, an AI-powered marketplace that delivers finance and accounting solutions to businesses through a combination of expert fractional talent, data-driven tools, and guiding insights. Eli has worked in the AI and machine learning field for over 10 years and served for five years as a limited term lecturer in the subjects of machine learning, data science, and AI at Purdue University.
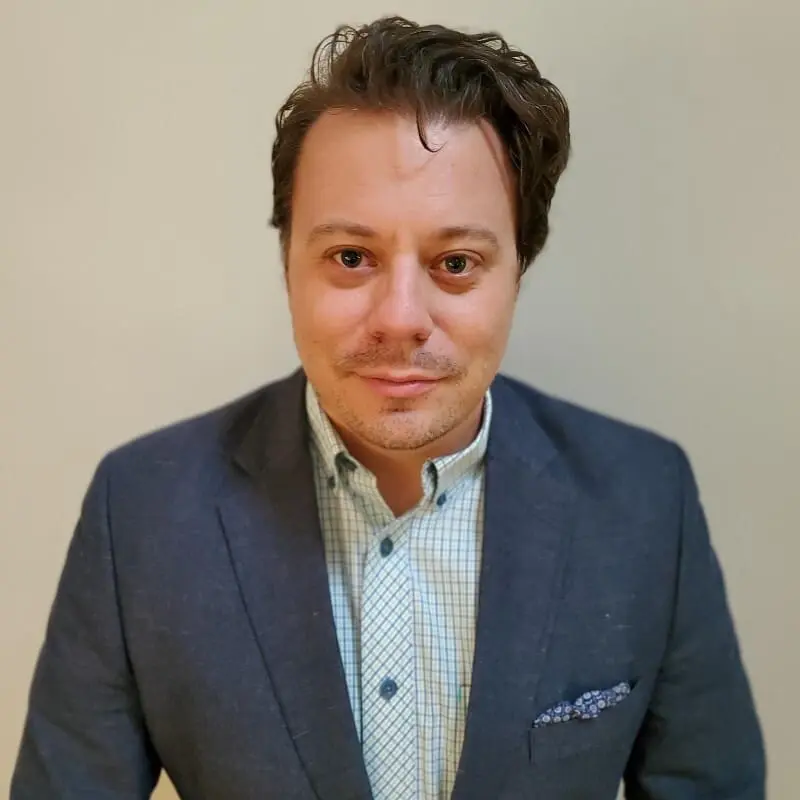