By Saum Mathur, COO, Paro
Over the past three years, AI spending projections for 2024 have doubled to over $200 billion. Organizations, governments and individuals are racing to explore the possibilities, unleashing a flood of ethical issues with AI and raising questions about how we can build inclusive, trustworthy models that serve all users and customers.
Solving this challenge brings three design priorities to the fore: mitigating bias, promoting explainability and approaching AI as a multiplier of human performance, not a replacement for it. Together, they flip the script on AI-human relationships. Training AI in this way requires us to check our biases, codify our values in code and ultimately perform at a higher level.
Inclusivity as the Foundation of Ethical and Effective AI
False imprisonment. Federal Trade Commission bans. Housing bias. These are examples of noninclusive AI applications failing their intended purposes and exacting harm on individuals, businesses and society.
Inclusivity creates better outcomes for all players, including businesses. By definition, it requires awareness of how our models directly and indirectly affect everyone involved. Given the rapidly changing dynamics of these issues, the path to inclusive AI is one guided by continuous learning and adaptation. It begins with three important stepping stones of understanding.
1. Bias: How It Causes, Perpetuates and Exacerbates Ethical Issues with AI
AI models become biased because they’re trained on datasets and processes that humans create—and humans carry societal and personal subjectivity into everything we do.
- Biased datasets: Using personal judgment, we decide on the goals, scope, questions, subjects, parameters and means of collecting data. Each decision can limit the completeness and representativeness of a dataset—i.e., its ability to accurately represent reality—often by omission or assumption. Those assumptions and gaps represent biases that then inform algorithmic decisions.
For example, after training on 10 years of resume submissions, mostly from men, Amazon’s recruiting algorithm learned to score men overwhelmingly higher than women, even disqualifying resumes mentioning “women”.
- Algorithmic bias: Humans also define how an algorithm applies data to its decision-making process, e.g., what’s relevant, what to exclude, what to favor in certain conditions, when to reward or penalize a recommendation based on feedback, how success is defined, etc. As a result, systemic, repeating errors that produce unfair outcomes can form and grow.
Say you create a compensation-recommendation algorithm based on performance that considers everyone’s performance data equally. A young person unburdened with childcare may work longer hours, resulting in higher performance than that of an older person with kids. The algorithm may learn to associate lower age with overperformance and higher age with underperformance, which isn’t accurate.
The consequences are most severe when biased results are reincorporated into the training model or added to new training datasets—thus perpetuating and amplifying the bias. While you can’t circumvent bias, you can follow these strategies to reduce it:
- Develop a strategic perspective at the outset. You can’t completely remove bias after it’s ingrained, so act early. Define the purpose of what you’re building, how it will be used, what data you’ll train it on and why.
- Be willing to recognize bias. Seek a diversity of opinion. invite stakeholders and domain experts to weigh in.
- Partition your data into separate subsets targeting each area of bias, then train and test performance for each one. Other techniques may include “cleaning” your data of bias, weighting it differently or training the model to remove points of bias.
- Continuously evaluate the results. How are they impacting various parties? Ensure that any results going back into the training model are providing equitable outcomes and monitor for emergent biases.
At my company, Paro, we wanted to proactively solve for potential biases in our freelancer-to-client matching algorithm so that new experts to our network would not be passed over experts with a successful track record in our network. To eliminate bias, our model suggests both seasoned and new experts. Clients unknowingly choose from the mix. Selected new candidates are then promoted in future recommendations, ensuring equal exposure opportunities based on client-chosen merit rather than arbitrary factors.
2. Explainability and Transparency in AI: Cultivating Trust
One of the biggest ethical issues with AI is the black box problem. According to a recent survey we conducted among finance executives, one third of financial professionals cite lack of transparency or understanding of how AI works as a top concern for adoption.
The inability to discuss how a model trains, makes decisions, arrives at conclusions and learns presents serious risks, particularly in the finance function. Without knowing which levers to pull, how can you:
- Tweak an intelligent forecasting model in light of an unprecedented event?
- Route capital with confidence?
- Determine accountability when problems arise?
- Ensure you’re maintaining compliance and company values?
- Stand up to auditor questioning?
- Identify and curb bias in your processes?
Most importantly, how do you make sure you’re still in control?
I practice the alternative: “white box” AI. I sit with stakeholders to explain how our algorithms work and why they’re trustworthy in plain English. What types of data is it learning from? What type of model is it and what are the key variables or features driving its outputs? What confidence metrics are we using? This is the minimum level of AI transparency you should expect from your vendor. They should also encourage you to challenge their model regularly.
3. Job Enhancement: What AI Taught Us About Amplifying Human Intelligence
According to surveyed finance professionals, the loss of judgment and human oversight is the second highest concern, just behind data security. Trepidation grows with title: more than half of VPs, managing directors, directors and divisional department heads ranked it as their top concern.
Yes, AI has the potential to cut certain jobs. But in practice, we’ve found we serve clients better by using AI as a multiplier of human intelligence and efficiency. Through continuous learning and adaptation, AI models can give us a deeper sense of what customers really want, offering an encouraging revelation: the things that make us human are valuable.
For example, an AI model might make predictions based on a specific set of assumptions about customer behavior, but user—or human—feedback may reveal more nuanced drivers and customer needs that, with model retraining, can further improve model accuracy. The ability for AI to continuously adapt its priorities based on emerging insights allows it to elevate areas where human judgment and relatability deliver greater value.
Judging by the proliferation of “cobot” or “copilot”-like products designed to complement human effort, we’re not alone in our belief that AI actually accentuates positive human characteristics while amplifying performance.
An Era of Innovation Enhanced by an Ethical Backbone
AI is the future of business. Finance teams stand to gain the most, using automation and AI to become powerful strategic partners.
The inflection point, however, is not rapid transformation but a commitment to serve people equitably. To that end, these priorities will help you embed diversity and empathy into your work and wield AI to empower people first.
Is your business considering AI adoption? Ensure AI readiness with the help of Paro. With decades of combined experience in implementing and driving value with AI, we’re here to help businesses identify the right path and course of action for success. Schedule a consultation today.
About the Author
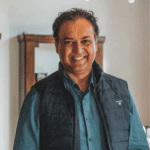
Saum Mathur has 29 years of experience in driving business growth by leveraging leading-edge business concepts, advanced analytics, and technologies. He is a progressive and results-oriented executive who thrives on challenges and opportunities. As the Chief Operating Officer at Paro, a platform that connects businesses with freelance finance and accounting experts, he leads the marketing, sales, product management, technology, and revenue operations for the company, with the mandate to grow the company efficiently and sustainably.